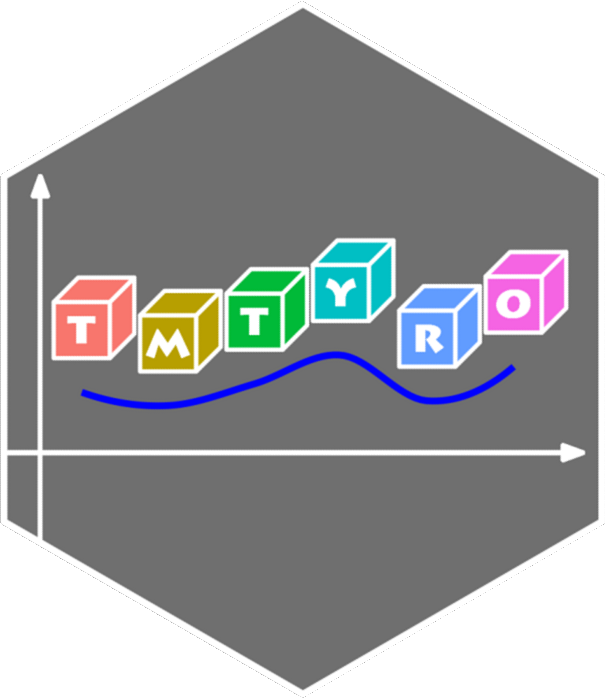
Ramping up skills
Stepping into dplyr
Source:vignettes/articles/03-skills-ramp.Rmd
03-skills-ramp.Rmd
tmtyro is optimized for a fast start, speeding users along a standard
workflow built on tidytext and the tidyverse. But it also serves as a
skills ramp, offering vectorized functions that work seamlessly with a
broader ecosystem of tools. These functions provide a stepping stone for
users transitioning to text mining workflows that leverage the
flexibility and power of dplyr. The vectorized functions outlined below
present an alternative pathway to the workflows enabled by functions
like add_frequency()
and add_sentiment()
,
bridging the gap to more advanced techniques.
Working with vectors
We often work with text as a vector of words, sometimes thought of as a “bag” of words. In this form, everything is typically standardized into lowercase spellings, punctuation marks are removed, and words are split at spaces:
library(stringr)
primer <- "See Jack run. Run, Jack! Run well!" |>
tolower() |>
str_remove_all("[:punct:]") |>
strsplit(" ") |>
unlist()
primer
#> [1] "see" "jack" "run" "run" "jack" "run" "well"
tmtyro offers functions for working with such lists or bags of words,
returning an equal-length list of some measurement or test. For
instance, get_frequency()
returns word counts for each word
in a list:
get_frequency(primer)
#> [1] 1 2 3 3 2 3 1
These counts can be converted to percentages by adding
percent = TRUE
or by using the shorthand
get_tf()
:
get_frequency(primer, percent = TRUE)
#> [1] 0.1428571 0.2857143 0.4285714 0.4285714 0.2857143 0.4285714 0.1428571
get_tf(primer)
#> [1] 0.1428571 0.2857143 0.4285714 0.4285714 0.2857143 0.4285714 0.1428571
Most of tmtyro’s functions for working with vectors begin with
get_...()
. This standardization helps to simplify
autocomplete. Additionally, two logical tests beginning with
is_...()
return values TRUE
or
FALSE
:
tmtyro function | returns | use | result |
---|---|---|---|
get_frequency() |
word frequencies as counts | get_frequency(primer) |
1 , 2 , 3 , 3 , 2 , 3 , 1 |
get_frequency(percent = FALSE) or get_tf() |
word frequencies as percentages | get_tf(primer) |
0.14 , 0.29 , 0.43 , 0.43 , 0.29 , 0.43 , 0.14 |
get_cumulative_vocabulary() |
cumulative count of new words | get_cumulative_vocabulary(primer) |
1 , 2 , 3 , 3 , 3 , 3 , 4 |
get_ttr() |
cumulative type-token ratio | get_ttr(primer) |
1 , 1 , 1 , 0.75 , 0.6 , 0.5 , 0.57 |
get_htr() |
cumulative hapax-token ratio | get_htr(primer) |
1 , 1 , 1 , 0.5 , 0.2 , 0.17 , 0.29 |
get_hir() |
hapax introduction rate | get_hir(primer) |
1 , 0.5 , 0.33 , 0.25 , 0.2 , 0.17 , 0.29 |
get_match() |
word matches according to a dictionary | get_match(primer, verbs) |
“yes” , NA , “no” , “no” , NA , “no” , NA |
get_sentiment() |
word matches according to a sentiment dictionary | get_sentiment(primer) |
NA , NA , NA , NA , NA , NA , “positive” |
is_new() |
whether a word is newly being added to the list | is_new(primer) |
TRUE , TRUE , TRUE , FALSE , FALSE , FALSE , TRUE |
is_hapax() |
whether a word is used only once in the list | is_hapax(primer) |
TRUE , FALSE , FALSE , FALSE , FALSE , FALSE , TRUE |
Working with and becoming familiar with these vectorized functions can be a useful step for gaining confidence in working with text data.
Working with tables
Although text analysis often conceives of documents as bags of words, tmtyro typically follows tidytext principals to go further, with data organized in a table with one word per row. This method of organization allows for both context, in rows above and below, and understanding, in columns to the left and right.
Adding columns
tmtyro’s typical workflow offers a selection of verbs for adding new
columns—all helpfully beginning with add_...()
.
Alternatively, the vector functions listed above work well with dplyr’s
mutate()
for adding columns:
# Load a corpus
corpus_dubliners <- get_gutenberg_corpus(2814) |>
load_texts() |>
identify_by(part) |>
standardize_titles() |>
select(doc_id, word)
# Use mutate() to add columns
corpus_dubliners |>
mutate(
count = get_frequency(word),
percent = get_tf(word),
new = is_new(word),
hapax = is_hapax(word))
#> # A tibble: 67,953 × 6
#> doc_id word count percent new hapax
#> <fct> <chr> <int> <dbl> <lgl> <lgl>
#> 1 The Sisters there 168 0.00247 TRUE FALSE
#> 2 The Sisters was 1161 0.0171 TRUE FALSE
#> 3 The Sisters no 168 0.00247 TRUE FALSE
#> 4 The Sisters hope 14 0.000206 TRUE FALSE
#> 5 The Sisters for 508 0.00748 TRUE FALSE
#> 6 The Sisters him 494 0.00727 TRUE FALSE
#> 7 The Sisters this 124 0.00182 TRUE FALSE
#> 8 The Sisters time 119 0.00175 TRUE FALSE
#> 9 The Sisters it 582 0.00856 TRUE FALSE
#> 10 The Sisters was 1161 0.0171 FALSE FALSE
#> # ℹ 67,943 more rows
Grouping documents
Not every measurement makes sense in a big corpus, where we might
care more about things on a document-by-document basis. For this reason,
tmtyro offers a few additional functions for measuring grouped values.
Functions of this type are named in a pattern using
get_..._by()
:
function | returns |
---|---|
get_tf_by() |
term frequencies (as a percentage) for each word by document |
get_idf_by() |
inverse document frequencies for each word by document |
get_tfidf_by() |
term frequency–inverse document frequencies for each word by document |
Because they expect two arguments of equal length, these kinds of
vector-document functions are best suited for data organized in a table.
All of these get_..._by()
functions use the same
syntax:
corpus_dubliners |>
mutate(
tf = get_tf_by(word, doc_id),
idf = get_idf_by(word, doc_id),
tf_idf = get_tfidf_by(word, doc_id))
#> # A tibble: 67,953 × 5
#> doc_id word tf idf tf_idf
#> <fct> <chr> <dbl> <dbl> <dbl>
#> 1 The Sisters there 0.00482 0 0
#> 2 The Sisters was 0.0180 0 0
#> 3 The Sisters no 0.00514 0 0
#> 4 The Sisters hope 0.000321 0.511 0.000164
#> 5 The Sisters for 0.0103 0 0
#> 6 The Sisters him 0.0138 0 0
#> 7 The Sisters this 0.00193 0.0690 0.000133
#> 8 The Sisters time 0.000964 0 0
#> 9 The Sisters it 0.0119 0 0
#> 10 The Sisters was 0.0180 0 0
#> # ℹ 67,943 more rows
Native to dplyr, group_by()
and ungroup()
offer another method for calculating values by document:
corpus_dubliners |>
group_by(doc_id) |>
mutate(
tf = get_tf(word)) |>
ungroup()
#> # A tibble: 67,953 × 3
#> doc_id word tf
#> <fct> <chr> <dbl>
#> 1 The Sisters there 0.00482
#> 2 The Sisters was 0.0180
#> 3 The Sisters no 0.00514
#> 4 The Sisters hope 0.000321
#> 5 The Sisters for 0.0103
#> 6 The Sisters him 0.0138
#> 7 The Sisters this 0.00193
#> 8 The Sisters time 0.000964
#> 9 The Sisters it 0.0119
#> 10 The Sisters was 0.0180
#> # ℹ 67,943 more rows
Be aware that that there’s no equivalent get_idf()
or
get_tfidf()
for pairing with group_by()
. By
definition, these calculations need contextual awareness of documents in
a corpus.
Using dplyr in this way allows for a range of measurements beyond those imagined in tmtyro. For instance, it might be helpful to express term frequencies in terms of how far they are from average use:
unique_usage <- corpus_dubliners |>
group_by(doc_id) |>
mutate(
tf = get_tf(word),
mean = mean(tf, na.rm = TRUE),
sd = sd(tf, na.rm = TRUE),
z_score = (tf - mean)/sd) |>
ungroup() |>
select(-c(mean, sd))
unique_usage
#> # A tibble: 67,953 × 4
#> doc_id word tf z_score
#> <fct> <chr> <dbl> <dbl>
#> 1 The Sisters there 0.00482 -0.396
#> 2 The Sisters was 0.0180 0.498
#> 3 The Sisters no 0.00514 -0.375
#> 4 The Sisters hope 0.000321 -0.702
#> 5 The Sisters for 0.0103 -0.0253
#> 6 The Sisters him 0.0138 0.215
#> 7 The Sisters this 0.00193 -0.593
#> 8 The Sisters time 0.000964 -0.658
#> 9 The Sisters it 0.0119 0.0838
#> 10 The Sisters was 0.0180 0.498
#> # ℹ 67,943 more rows
In this way, one could find outlier words by defining them as being at least a standard deviation away from standard usage in a document.
unique_usage |>
select(doc_id, word, z_score) |>
filter(abs(z_score) >= 1) |>
distinct()
#> # A tibble: 54 × 3
#> doc_id word z_score
#> <fct> <chr> <dbl>
#> 1 The Sisters the 3.01
#> 2 The Sisters i 1.31
#> 3 The Sisters and 1.85
#> 4 The Sisters to 1.33
#> 5 An Encounter the 3.06
#> 6 An Encounter to 1.08
#> 7 An Encounter he 1.39
#> 8 An Encounter of 1.14
#> 9 An Encounter and 1.52
#> 10 Araby the 2.95
#> # ℹ 44 more rows
This particular example unhelpfully emphasizes stopwords, but the process models the kind of checking that often proves helpful when working with text.
Getting representational data
Instead of studying every word in context, it’s sometimes helpful to
get representational data from each document. Standard dplyr functions
helpfully limit groups to subsets of rows. For instance,
distinct()
drops any rows that repeat:
# mutate() keeps every row
simple_freq <- corpus_dubliners |>
group_by(doc_id) |>
mutate(tf = get_tf(word)) |>
ungroup()
# distinct() keeps the first instance of each row
simple_freq |>
distinct()
#> # A tibble: 17,692 × 3
#> doc_id word tf
#> <fct> <chr> <dbl>
#> 1 The Sisters there 0.00482
#> 2 The Sisters was 0.0180
#> 3 The Sisters no 0.00514
#> 4 The Sisters hope 0.000321
#> 5 The Sisters for 0.0103
#> 6 The Sisters him 0.0138
#> 7 The Sisters this 0.00193
#> 8 The Sisters time 0.000964
#> 9 The Sisters it 0.0119
#> 10 The Sisters the 0.0549
#> # ℹ 17,682 more rows
Combining elements of both mutate()
and
distinct()
, the summarize()
function returns
one row per group while allowing new column definitions:
# summarize() returns one row per group, here the maximum tf value for combination of doc_id and word
simple_freq |>
group_by(doc_id, word) |>
summarize(tf = max(tf)) |>
ungroup()
#> # A tibble: 17,692 × 3
#> doc_id word tf
#> <fct> <chr> <dbl>
#> 1 The Sisters 1895 0.000321
#> 2 The Sisters 1st 0.000321
#> 3 The Sisters a 0.0148
#> 4 The Sisters about 0.00353
#> 5 The Sisters above 0.000321
#> 6 The Sisters absolve 0.000321
#> 7 The Sisters acquaintance 0.000321
#> 8 The Sisters acts 0.000321
#> 9 The Sisters added 0.000321
#> 10 The Sisters advertisements 0.000321
#> # ℹ 17,682 more rows
Offering a little more flexibility, dplyr’s slice_...()
family of functions is helpful for choosing a subset of rows in each
group. For instance, slice_max()
makes it easy to get the
top 3 words used in each story from Dubliners, ordered by term
frequency:
simple_freq |>
distinct() |>
group_by(doc_id) |>
slice_max(
order_by = tf,
n = 3) |>
ungroup()
#> # A tibble: 48 × 3
#> doc_id word tf
#> <fct> <chr> <dbl>
#> 1 The Sisters the 0.0549
#> 2 The Sisters and 0.0379
#> 3 The Sisters to 0.0302
#> 4 An Encounter the 0.0556
#> 5 An Encounter and 0.0329
#> 6 An Encounter he 0.0310
#> 7 Araby the 0.0810
#> 8 Araby i 0.0409
#> 9 Araby and 0.0299
#> 10 Araby to 0.0299
#> # ℹ 38 more rows
Other slicing functions like slice_sample()
and
slice_head()
offer additional options for preparing data to
study.
Dictionary matching
Many of the vectorized get_...()
functions return
numeric or logical results, but two return values based on a dictionary
of terms. These functions are perhaps best demonstrated with a new bag
of words:
primer2 <- "Jack hates rainy days." |>
tolower() |>
str_remove_all("[:punct:]") |>
strsplit(" ") |>
unlist()
The more general get_match()
function returns the
dictionary match for any word or vector. When paired with a dictionary
made with make_dictionary()
, it returns values for any
matches found:
emoji_weather <- make_dictionary(
list(
"️☔️" = c("rain", "rains", "rainy", "raining"),
"️⛈️" = c("storm", "storms", "stormy", "storming"),
"☁️" = c("cloud", "clouds", "cloudy"),
"🌞" = c("sun", "sunny"),
"🌫️" = c("fog", "fogs", "foggy", "mist", "misty"),
"🌬️" = c("wind", "winds", "windy"),
"️❄️" = c("snow", "snows", "snowing")),
name = "weather")
primer2
#> [1] "jack" "hates" "rainy" "days"
get_match(primer2, emoji_weather)
#> [1] NA NA "️☔️" NA
As shown here, unmatched values return NA
missing
values.
The function works with dplyr’s mutate()
much like any
other vectorized function:
dubliners_weather <- corpus_dubliners |>
mutate(weather = get_match(word, emoji_weather))
dubliners_weather |>
# show only one story and skip a few hundred words
filter(doc_id == "The Dead") |>
filter(row_number() > 609)
#> # A tibble: 15,122 × 3
#> doc_id word weather
#> <fct> <chr> <chr>
#> 1 The Dead he NA
#> 2 The Dead stood NA
#> 3 The Dead on NA
#> 4 The Dead the NA
#> 5 The Dead mat NA
#> 6 The Dead scraping NA
#> 7 The Dead the NA
#> 8 The Dead snow ️❄️
#> 9 The Dead from NA
#> 10 The Dead his NA
#> # ℹ 15,112 more rows
dubliners_weather |>
drop_na()
#> # A tibble: 55 × 3
#> doc_id word weather
#> <fct> <chr> <chr>
#> 1 The Sisters clouds ☁️
#> 2 The Sisters sunny 🌞
#> 3 The Sisters sun 🌞
#> 4 The Sisters clouds ☁️
#> 5 An Encounter storm ️⛈️
#> 6 An Encounter sunny 🌞
#> 7 An Encounter sun 🌞
#> 8 An Encounter clouds ☁️
#> 9 Araby rainy ️☔️
#> 10 Araby rain ️☔️
#> # ℹ 45 more rows
Matching sentiment
A sentiment lexicon is just a special kind of dictionary. To support
a specialized case for sentiment analysis, get_sentiment()
works the same way as get_match()
:
primer2
#> [1] "jack" "hates" "rainy" "days"
get_match(primer2, tidytext::get_sentiments("bing"))
#> [1] NA "negative" NA NA
get_sentiment(primer2, "bing")
#> [1] NA "negative" NA NA
Like other functions, it works well with dplyr’s
mutate()
for adding a column of sentiment
interpretation:
corpus_dubliners |>
mutate(
sentiment = get_sentiment(word, "bing")) |>
drop_na()
#> # A tibble: 3,867 × 3
#> doc_id word sentiment
#> <fct> <chr> <chr>
#> 1 The Sisters evenly positive
#> 2 The Sisters dead negative
#> 3 The Sisters darkened negative
#> 4 The Sisters blind negative
#> 5 The Sisters idle negative
#> 6 The Sisters strangely negative
#> 7 The Sisters like positive
#> 8 The Sisters like positive
#> 9 The Sisters sinful negative
#> 10 The Sisters fear negative
#> # ℹ 3,857 more rows
By combining simplicity and flexibility, tmtyro helps users move between streamlined workflows and customizable approaches. Whether working with small text datasets or exploring complex corpora, vectorized functions offer a bridge to mastering tools like dplyr. This approach encourages skill development in text mining, data analysis, and broader R programming techniques.